Harnessing the Power of Machine Learning for Email Spam Filtering
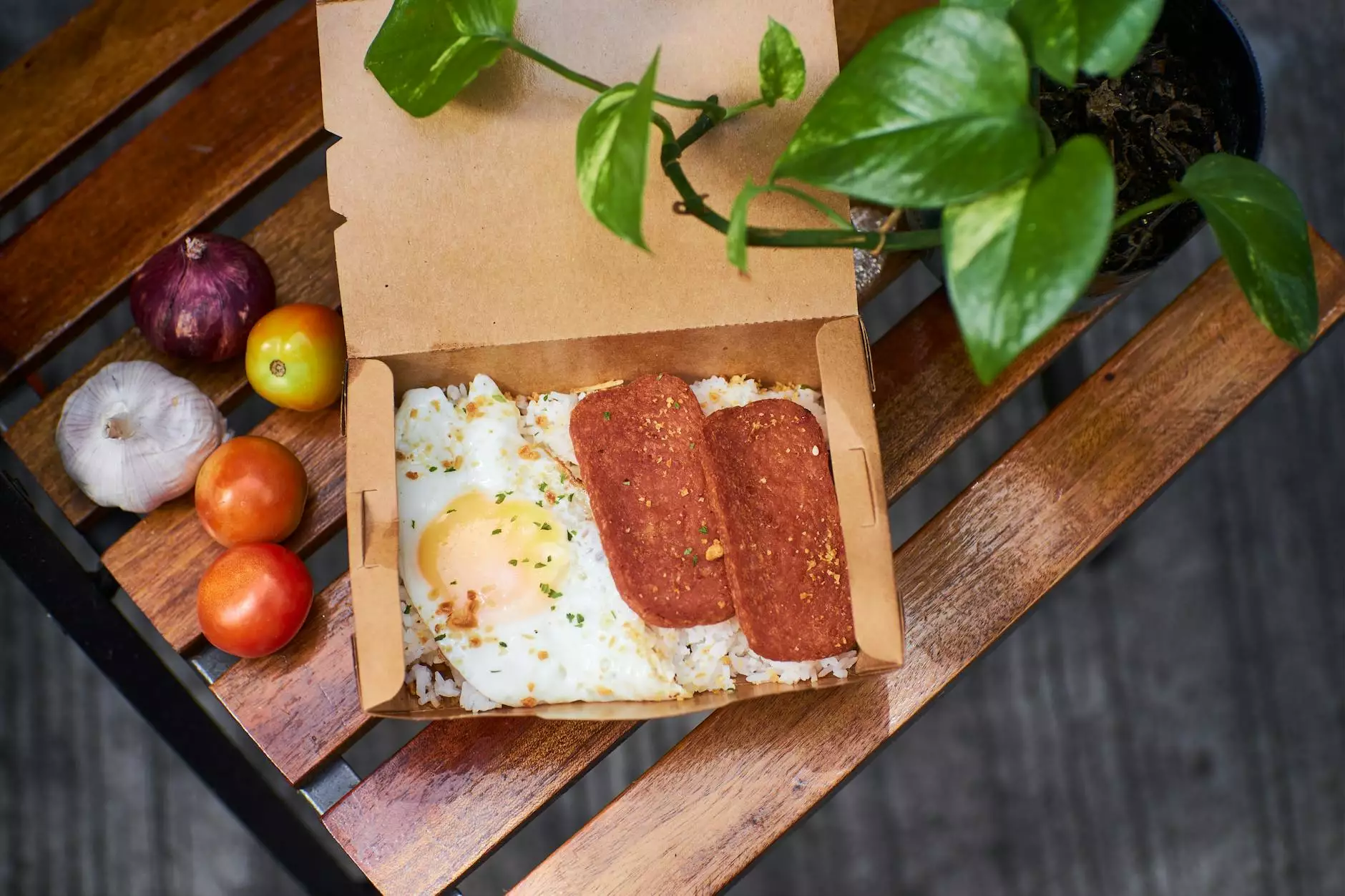
Introduction to Email Spam Filtering
Email has become a critical component of modern business communication. However, with the growing use of emails, the threat of spam has also surged. Spam emails not only clutter our inboxes but can also pose significant security risks, including phishing attacks and malware distribution. As organizations strive to maintain effective communication practices, machine learning for email spam filtering emerges as a robust solution to combat these challenges.
What is Machine Learning?
Before delving into spam filtering, it is essential to understand what machine learning (ML) entails. Machine learning is a subset of artificial intelligence (AI) focused on developing algorithms that improve automatically through experience. In simpler terms, ML enables software applications to become more accurate at predicting outcomes without being specifically programmed for those tasks.
Why Use Machine Learning for Email Spam Filtering?
Traditional spam filtering methods often rely on static rules and keyword blacklisting. While they can be somewhat effective, they are inadequate against evolving spam tactics. This is where machine learning for email spam filtering shines. Here are several reasons why businesses should consider this approach:
- Dynamic Learning: ML algorithms learn from new data, resulting in continually evolving filters that adapt to emerging spam tactics.
- Higher Accuracy: By analyzing patterns in large datasets, machine learning models can distinguish between legitimate emails and spam with greater precision.
- Reduced False Positives: Machine learning minimizes the risk of incorrectly labeling legitimate emails as spam, ensuring critical communications reach their intended recipients.
- Scalability: As your organization grows, so does your email traffic. Machine learning models can scale according to the data volume without substantial manual adjustments.
How Machine Learning Works in Email Spam Filtering
Machine learning for email spam filtering typically involves three key processes: data collection, training the model, and implementation.
1. Data Collection
The initial step involves aggregating a comprehensive dataset that includes both spam and non-spam emails. Each email is often classified based on various features, such as:
- Content of the email (text, images, hyperlinks)
- Sender's email address and domain
- Email structure and formatting
- Historical interaction patterns of the recipient with similar emails
2. Training the Model
Once the data is collected, it is used to train a machine learning model. This process involves feeding the model numerous examples of both spam and legitimate emails. Common algorithms used include:
- Naive Bayes Classifier: Applies Bayes' theorem to predict the likelihood of email being spam based on its content.
- Support Vector Machine (SVM): Works to find a hyperplane that best separates spam from non-spam emails.
- Neural Networks: Mimics the human brain's interconnected neuron structure, allowing for complex pattern recognition.
3. Implementation and Continuous Learning
After training, the model is implemented into the email system, where it can classify incoming messages. Continuous learning is vital; as new spam tactics arise, the model should refine itself by learning from newly labeled emails. This iterative learning process helps maintain high accuracy rates.
Benefits of Utilizing Machine Learning for Your Business
Incorporating machine learning for email spam filtering into your business not only enhances your email security but also offers a multitude of benefits:
- Enhanced Security: Prevents potentially dangerous spam emails, thereby safeguarding your sensitive information.
- Increased Productivity: By filtering out spam effectively, employees can focus on their work without distractions, improving overall productivity.
- Cost-Effectiveness: Reduces the time and resources spent managing spam-related issues, resulting in significant cost savings.
- Improved Customer Relationship Management: Ensures that important communications from clients or partners are never missed or misclassified.
Challenges of Machine Learning in Spam Filtering
Despite the numerous advantages, machine learning models also face challenges that organizations must acknowledge:
- Data Quality: ML algorithms require high-quality, diverse training data to function optimally. Inaccurate or biased datasets can lead to poor classification results.
- Overfitting: If a model is too complex, it may learn the training data too well, resulting in poor performance on new, unseen emails.
- Resource Intensity: Training machine learning models can be resource-intensive, requiring significant computational power and time.
- Maintaining Model Relevance: Continuous updates to the training dataset are necessary to keep the model relevant against evolving spam tactics.
Best Practices for Implementing Machine Learning for Email Spam Filtering
To successfully harness machine learning for email spam filtering, enterprises should consider the following best practices:
1. Utilize Robust Data Sources
Ensure your training data includes a wide variety of email types. Consider sourcing emails from various industries and regions to achieve a well-rounded model.
2. Regularly Update Your Models
Continuously refine your models based on new data and user feedback. This ensures the model adapts to changing spam tactics and stays efficient.
3. Monitor Performance Metrics
Use relevant performance metrics, such as false positive rates and recall rates, to assess the effectiveness of your spam filters. This will aid in making data-driven improvements.
4. Combine with Traditional Methods
Consider complementing ML approaches with traditional spam filtering methods for a layered defense mechanism. This hybrid approach often yields better results.
Conclusion
The digital landscape is constantly evolving, and so are threats to email security. By implementing machine learning for email spam filtering, organizations can effectively protect themselves against spam while ensuring that genuine communications are prioritized. By leveraging this innovative technology, businesses can not only enhance their operational efficiency but also improve overall security posture.
Contact Us Today
If you're ready to take your email security to the next level, Spambrella is here to help. Our expertise in IT Services & Computer Repair and Security Systems ensures that we can provide you with tailored solutions that fit your needs. Contact us today for a consultation on how we can implement cutting-edge machine learning solutions for your email spam filtering needs.